Methodological proposal for measuring and
predicting Urban Green Space per capita in a Land-Use Cover Change
model: Case Study in Bogota
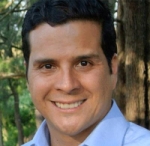 |
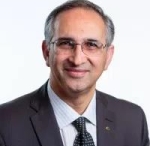 |
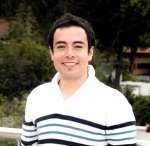 |
Daniel Páez |
Abbas Rajabifard |
Joaquín Andrés Franco Gantiva |
This paper was presented at
the Commission 7 Annual Meeting in Bogota Colombia. It describes the
problem of lack of sustainable urban planning and territorial ordinance
plans which have led to nullification, fragmentation and reduction of
green space and strategic ecosystems within cities.
SUMMARY
The lack of sustainable urban planning and territorial ordinance
plans have led to the nullification, fragmentation and reduction of
green space and strategic ecosystems within cities. A clear example of
this problem is the city of Bogotá. Currently, Bogota has around 4 m2 of
green space (GS) per capita, an amount significantly below the 10 m2
recommended by the World Health Organization (WHO). This research aims
to establish how GS is distributed and relates to other land uses,
transport infrastructure and other variables. For this purpose, ordinary
least squares (OLS) and geographic regression (GWR) models were used.
Both models are intended to determine the relationship at global and at
neighbourhood scale between the GS per capita and other variables. Using
Metronamica software and Information from 2005 and 2014, a 2040 greener
scenario for the city of Bogota was developed. Results from this
research highlighted the location of green space problems in Bogota. It
has also opened the opportunity for decision-makers to understand in a
quantitative way how GS is distributed and its benefits. Improve GS
relationships is expected to support sustainable planning in large
cities.
1. INTRODUCTON
Since the industrial revolution, most of the population growth has
been concentrated in the cities. They have become supports of the modern
economy and the centre of development for any country. This type of
artificial ecosystem is characterized by their changeable and dynamic
system that daily are consuming, transforming and releasing materials
and energy (Bottalico, et al., 2016). This dynamism is correlated with
increased production and consumption of goods, services and
infrastructure. However, at the same time, it has led to greater land
urbanization, landscape fragmentation, biodiversity loss, the creation
of urban heat islands, increasing greenhouse gas emissions, increasing
vulnerability to climate change events and the destruction of strategic
or sensitive ecosystems (Norton, et al., 2015).
To reduce and overcome these issues many cities, from developed and
developing countries, are evaluating mechanisms to plan and structure a
more efficiently growth. Commonly four main principles are use: economic
development, environmental equilibrium and sustainable development,
transparent policies and government, and are focusing on cultural
identity, factors that can be evaluated and grouped in a sustainability
circle (James, Magee, Scerri, & Steger, 2015). This new city planning is
becoming a stronger movement mainly in cities of developed countries,
where planning policies are focused towards sustainable modes of
transport, more compact and dense cities, efficient, greener and
liveable for current and future generations (Gedge, 2015) & (Barbosa, et
al., 2007) & (Demuzere, et al., 2014).
One of the main pillars of this new wave of urban planning thinking
is the development towards environmental sustainability. This can be
seen in several cities, mainly Europe and Asia (e.g. Vitoria-Gasteiz or
Singapore). In this cities, they have focused their attention on
generating and preserving all the potential of green and blue spaces
(water bodies), in order to increase the quality of life of their
citizens (Jim C. Y., 2004). This planning thinking enables Urban Green
Infrastructure (UGI) to arise and can be interpreted as a hybrid
infrastructure between old and new buildings with Green Space
(henceforth as, GS) inside or in the border of the cities.
The UGI's have emerged as a tool to generate new GS or even to
recover those that were lost. In turn, it has proven the benefits that
this type of spaces provide, as they are not only part of the landscape,
but also at the level of ecosystem services, valuation and capture of
land value, tourism attractiveness, among others (Norton, et al., 2015)
(Bolund & Hunhammar, 1999) (Jim & Chen, 2006). Also, these arise as an
option in cities that are already consolidated and have a high
population density, but lack GS or have a decrease of green areas. Given
these conditions, these cities have a major vulnerability to the effects
of climate change. The lack of these strategical areas generates a
reduction in the land capacity of infiltration, changes in the
underground water flow, an increase of removal in mass events and of the
urban heat, a lack of tools to reduce or control air pollution, among
others (Jo, 2002).
Furthermore, all the planners and the decision makers must take in
consideration of the preservation and the greening of the city as a
priority urban planning; not only to understand the causes and impacts
which the urban sprawl brings but also the opportunities that new spaces
can provide. Therefore, the current research aims to understand GS
location based on its relationship with other land uses, transportation
infrastructure, water bodies and other variables. Also, it is desired to
identify which zones in a city lack of these spaces, in order to take
actions to generate new green infrastructure, mainly parks, in these
zones. The testing of this indicator will be applied in an existing
model called “Bogotá Land Development (BoLD)” which was built by the
group SUR in association with the French Development Agency (AFD, by
their acronyms in Spanish and French) and uses a dynamic land cover
change model based on a cellular automata (CA) software Metronamica.
2. BACKGROUND
Rapid urbanization in the last century has led to changes in GS
inside or near the city. Urbanization has contributed to the
disappearance of these spaces and ecosystems that have generated
significant changes in the urban climate dynamics. Today natural land
surfaces and urban vegetation has been replaced by surfaces and
constructions that have the ability to absorb solar radiation. This has
led to the generation of different microclimates in urban areas (Norton,
et al., 2015). The lack of GS led to substantial changes in the
infiltration and retention capacity of the soil, altering the flow of
groundwater (Demuzere, et al., 2014). These changes have led to a
gradual increase of temperature in urban areas, which is reflected by an
increase in forest fires events, landslides and the reduce disposal of
urban water resources, that finally leads to an increased vulnerability
for climate change at local level.
At the same time, urbanization patterns are always associated with
the fragmentation and nullification of strategic ecosystems. This not
only affects urban biodiversity and lack of open GS but also generates
changes in the city interactions with the environment and its surrounds
(Wu, 2014). Decreases of green areas mean greater vulnerability to
climate change event at the local scale (Yin, Olesen, Wang, Ozturk,
Zhang, 2016) (Duh, Shandas, Chang, & George, 2008). In order to address
and mitigate all the problems described above, cities are taking actions
such as improvement of infrastructure (Connop, et al., 2016) looking for
more sustainable and efficient transportation, reducing energy
consumption and even make the cities greener (Wolch, Byrne, & Newell,
2014). All of these without affecting the population growth and economic
development. One of the mechanisms that concentrate most of these
actions is the development of UGIs.
A UGI can be defined as a planned or unplanned GS, spanning both the
public and private realms, and managed as an integrated system to
provide ecosystem services (Norton, et al., 2015). The UGI is composed
of infrastructure that has native vegetation, parks, private garden,
golf courses, street trees, green roofs, green walls, biofilters, rain
gardens, wetlands, riparian zone and urban forest (Pakzad & Osmond,
2016). UGIs have a significant ecological, social and economic
functions, and at the same time has been indicated as a promising
infrastructure with the capacitive of reducing the adverse effects of
climate change in urban areas (Pakzad & Osmond, 2016).
There are a lot of benefits and ecosystem services with UGIs such as
sequester carbon dioxide emissions, purifying the air by producing
oxygen (Jo, 2002), regulate the micro-climate (Norton, et al., 2015),
reduce noise (Bolund & Hunhammar, 1999), protect soil and water (Pauleit
& Duhme, 2000), purifying and controlling the underground and
superficial water bodies (Pauleit & Duhme, 2000), maintained the
biodiversity (Attwell, 2000). Also, living nearby GS can make the sale
prices of properties increase considerably (Jim & Chen, 2006) also
contributes to public health (Tzoulas, et al., 2007) and increase the
quality of life of urban citizen (Barbosa, et al., 2007).
The concept of UGI is being implemented as part of future land use
plans in cities around the world. Most of the cities where this type of
infrastructure is developed, planned or implemented are the highly dense
and compact cities of the globe (Jim & Chen, 2003). Today’s urban size
is not a limitation when it comes to planning a greener city. This is
based on the fact that access to technologies of information and
communication (TIC), as well as the improvement of human understanding
around sustainability, have made it possible to promote cities that are
much more intelligent and efficient, main pillar of the Smart City
definition (Bibri & Krogstie, 2017) (Anguluri & Narayanam, 2017). This
concept has led to a reversal of the way society thinks in order to
obtain equilibrium in all populated centres and all the uses and
ecosystems that form it (Zuccalà & Verga, 2017). That is why there are
examples around the globe that demonstrate that cities that increase the
GS are the ones that are generating a set of public policies to become
greener, more resilient and efficient; while at the same time are really
compact and dense (Jim C. Y., 2004).
Barcelona and Medellin, are one of the few examples of compact cities
with the aim to recover and obtain more green areas and also be more
liveable and sustainable for their citizens. In the case of Barcelona,
the city has made a proposal plan to become a more green and biodiverse
city by 2020, the project pursuit to generate a genuine network of GS by
bringing nature into the city with all the life forms in order to make
it more fertile and resilient to the pressure and challenges of climate
change (Ayuntamiento de Barcelona, 2011).
While Medellin has developed a land use plan called BIO 2030. In this
plan, a 30 km linear park is planned in the river bank and next to it
densification in height, which aims to reduce the informal settlements,
recover mountain ecosystems, increase accessibility to public
infrastructure and public transportation located near the riverbank
(Alcaldía de Medellín, 2011).
Both plans were planned and conceived to allow these cities to be
more resilient to climate change events. Likewise, their
conceptualization started with the construction of sustainability
indicators that allow them to assess the feasibility of plans and
studies. Therefore, UGI’s must be based on indicators that allow them to
determine which principles ruled them and also how they interact with
their surrounds. These infrastructures must be related somehow to other
land uses and transportation networks.
2.1. Existing methodology for measure Urban Green Space (UGS)
A Lot of international and regional organization, such as the
European Foundation (EF), the European Commission on Science, Research
and Development (ECSRD), the United Nations (UN), the European
Commission on Energy Environment and Sustainable Development and the
World Bank have development a list of urban sustainability indicators
(Barbosa, et al., 2007) to emphasize the important of preserve and
increase the GS in the cities. In general, these indicators are
conceived to synthesized factors that affect the quality of life
including personal, social, cultural, community, natural environmental
and economic factors. Also. Parisa Pakzad and Paul Osmond (Pakzad &
Osmond, 2016), set and create a total of 30 indicators and then resume
in 9 major concepts that must be evaluated for every UGI; furthermore,
they reclassify in three categories: economic growth, environmental
sustainability and health & wellbeing.
Some studies show that there are different mechanisms to evaluate the
spatial distribution of parks, as well as the GS per capita in cities.
An investigation by Fan, Xu, Yue, & Chen (2016) analyses the spatial
distribution of parks from a green accessibility indicator (GAI). This
indicator is constructed from two perspectives: the first by means of an
expert survey to evaluate variables of services and nature from a
quantitative point of view. The second, by measuring the afferent
service area of the GS. This latest indicator is calculated using the
distances and methodology established by the standards of Accessibility
to Natural Green Space (ANGSt). Another methodology proposed by Gupta,
Roy, Luthra, Maithani, & Mahavir (2016) uses a GIS-based network to
analyse the accessibility of UGS. They took on account children
populations and socioeconomic groups near the UGS.
Another approach (de la Barrera, Reyes-Paecke, & Banzhaf, 2016)
quantifies the public space required by the inhabitants of Chinese
cities through a metric quantification defined by the area of GS
multiplied by corrected coefficients of quality and accessibility,
obtained a measure of GS that was called effective equivalent green
(EGE).
Another research methodology (Gupta, Kumar, Pathan, & Sharma, 2012)
measured the proximity to green, built up density, and the height of
structures in order to obtain an Urban Neighbourhood Green Index (UNGI).
These studies demonstrated how important GS are, as well as all the
ecosystem benefits and services they provide. However, questions remind
on their application in developing countries including how they are
distributed and the variables that affect their location over time.
To address this, the study of He, Li, Yu, Liu, & Huang (2017) urban
growth in Wuhan city, PR China, was evaluated through variables that
change over time. In this study, it is observed how one variable can
directly affect the geospatial location of the other. Transport
infrastructure and the built-up area sizes were identified as the
variables that affected most urbanization.
Several cities of the world are being planned to understand the
dynamics that exist in it, in order to attend their needs. Jianhua He
determined that the use of spatial interaction it is fundamental, in
order, to understand urban agglomeration system (He, Li, Yu, Liu, &
Huang, 2017). Likewise, (Zeng, Zhang, Cui, & He, 2015) establishes that
the new urbanizations are integrating the remote sensors, the spatial
analyses and the spatial geographic information to have a global vision
of the urbanization. In the case of Jianhua He, the variables for
quantifying urbanization came from three different categories: economic,
social and environmental, and it uses a spatially explicit approach
based on data field to analyse the spatial interaction in the Wuhan
city, PR China, through the regional transport infrastructure.
Meanwhile, Zeng suggested that the urban expansion was studied by
measuring 20 variables divided into three groups: characteristics,
density and proximity. The first study suggests a strong relationship
between the urban growth and distribution of uses linked to the
transportation infrastructure. Nonce Jianhua suggested the effect that
transportation has on the built-up area, and urbanization, all of it
obtained through spatial regression models. Finally, the above suggests
the importance of understanding the dynamics and interactions within
cities. And with the help of spatial analysis and regression been able
to understand that phenomena and with it being capable of a better,
greener and sustainable long-term city planning.
3. METHODS
3.1. Study area
Bogotá is the main political, economic, social and cultural centre of
Colombia. The city had a population that exceeded the 7.7 million
inhabitants in 2014, which corresponds approximately to 24 percent of
the entire population of the country (Munoz-Raskin 2010). In addition,
it is responsible for generating more than 25% of the National GDP (DANE
2015). Since 1991, Bogota gained the political status of “Capital
District” which allows the city to be governed independently from the
politics of the state. In other terms. Bogotá has autonomy in terms of
land – use planning, taxation, independent cadastre, infrastructure
development and management.
The city extends across 355 urban square kilometres (Bocarejo et al.
2013), limited in the east by mountains, in the south by the Sumapaz
Moorland; on the western edge lies the Bogotá river and in the north by
the municipalities of Chía and Sopo. Its density is near 20,500
inhabitants per square kilometre (Bocarejo, Portilla Pardo, 2013).
Nowadays the city counts with a total of 96 metropolitan parks according
to with the IDRD (Instituto de Recreación y Deporte Distrital, acronym
in Spanish) (Scopellieti, et al., 2016). However, even though the city
has this number of metropolitan parks, the amount of GS per capita it is
just 4.10 m2, an amount significantly below the 10 m2 recommended by the
WHO (Scopellieti, et al., 2016), (Castillo, 2013) (WHO) All of these
implies that Bogotá, is a very compact city with very high density that
has a lack of UGS.
3.2. Data acquisition
All data used in this research is based on the model “BoLD”, which is
a LUCC model conducted for Bogotá and six bordering municipalities:
Cota, Facatativa, Funza, Madrid, Mosquera and Soacha. It was created in
order to understand and simulate the consequences that the development
of transport infrastructure projects has in the city, and their land
uses. The model was as part of a technical cooperation between the group
SUR of The University of Los Andes, and the French Development Agency
(AFD, for their acronyms in French and Spanish).
The study zone for BoLD project was divided into vacant (land uses
that are available and can be occupied by other land-uses), feature
(which are the land-uses that doesn’t have changes over the time) and
function (which corresponds to the land-uses that are in constant
change), all of them in a raster data resolution of 100m x 100m. Once
the land-uses and transport accessibility where defined, the suitability
zones, the zoning, the future land demands and the neighbourhood
relationship between the land-uses allowed to create a different dataset
for two different years: a baseline year (2005) and the calibration year
(2014). Once the calibration was completed, the model was used to
simulate and predict the LUCC from 2014 to 2040 in four different
scenarios of transport infrastructure and natural reserve conservation
(Paez & Escobar, 2016).
3.3. Methodological Framework
For this research, the land-use maps of the years 2005 and 2014 were
taken as the baseline for the architecture of the model. From these
datasets, the land-uses that correspond to residential, industrial,
commercial, wetland and equipment (the parklands were contained within
it) were extracted. With these layers, it was necessary to process all
this data in order to obtain the definitive shapefiles that will be used
in the model. Because the GS of the city was mixed with the equipment,
it was necessary to take the shapefile of parks, pass it to raster and
overlay it with the equipment of each year to obtain thus the GS
corresponding to 2005 and 2014 in the model BoLD, at the end the minimum
area obtained for a cell of GS was of one ha, which correspond to the
minimum size to evaluated those space as its suggested by different
international standards of the public GS (Natural England, 2010), (New
Yorkers for Parks, 2010) & (Force, 2002). It is important to establish
that for current research, GS correspond to parks and wetlands that are
in the urban area of the city.
An exploratory regression was crucial to identify which
variables could be most suitable for all the rules of an OLS regression.
After evaluating a series of possible combinations, an appropriate
predictive model to explain the phenomenon was found. In this research,
we assume diverse demographic, socioeconomic and environmental variables
for its association with the distribution of the public GS in Bogota. In
order to evaluate the model, a total of eighteen variables were
considered, the information of which was available for 2014. In the end,
only six variables were accepted, the remainder were discarded due to:
- Lack of information in the past and uncertainty of predicting in
the future.
- Redundancy with other variables or not an explanatory one,
cause it is a product of the dependable variable.
- Impossible to evaluate in a future scenario (due to the climate
and geographical conditions).
- They are variables that are very subjective and depend on an
applied survey to an expert or the community.
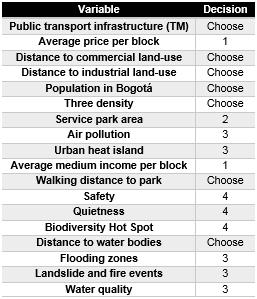
Table 1: Variables that
were considered at the beginning for the
development of the UGGI
Once the explanatory variables were identified and chose, an OLS
model was used to evaluate the linear relationship between the six
independent variables with the dependable variable, in order to obtain a
global model of the variable. These same steps have been performed in
previous research (Anderson, et al., 2014). It was found that the
equation that described the regression model is:

Equation 1. OLS regression model representation
Where y correspond to the GS per capita, over time; 𝛽0
correspond to the intercept of the model, 𝛽1
till 𝛽𝑛 represent the
coefficient of each independent variables, same as, 𝑋1
till 𝑋𝑛 which are the value of the explanatory variable; meanwhile the
𝜀 correspond to the residuals of the whole model.
This method assumes linearity in the model and the constant variant.
However, spatial data do not always fulfil all the presuppositions that
this method of regression requires (He, Okada, Zhang, Shi, & Li, 2008) &
(He, Zhang, Shi, Okada, & Zhang, 2006). In any case, if this method is
executed in conjunction with spatial autocorrelation, it could determine
if the variables are statistically significant and the model is well
specified and has been implemented properly. The Figure 1 and Figure 2
correspond to the spatial distribution of the six explanatory variables
chosen for 2005 and 2014.
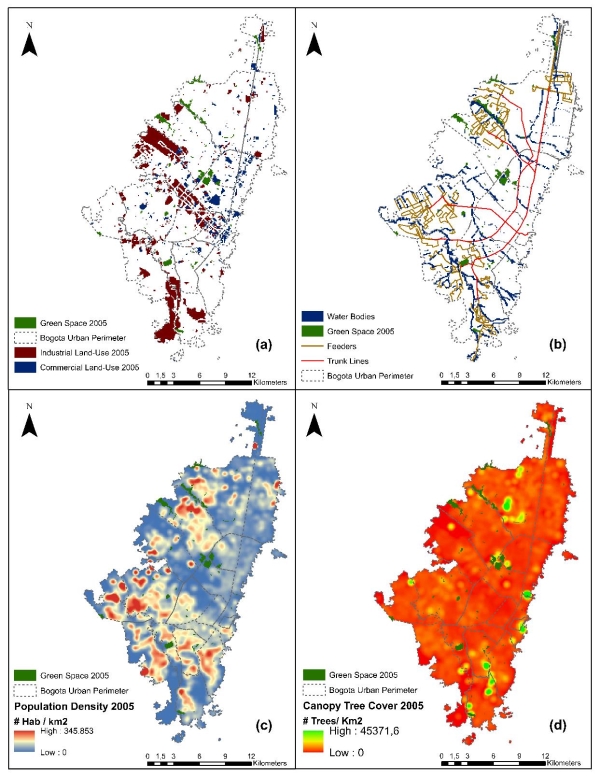
(a): Commercial and Industrial Land-use (b):
Public transportation network (c): Population Density (d): Canopy tree
cover
Figure 1. Spatial distribution of the population
density, canopy tree density cover, public transportation network, water
bodies, urban green space, commercial and industrial land uses in Bogotá
in 2005, 2014 and 2040
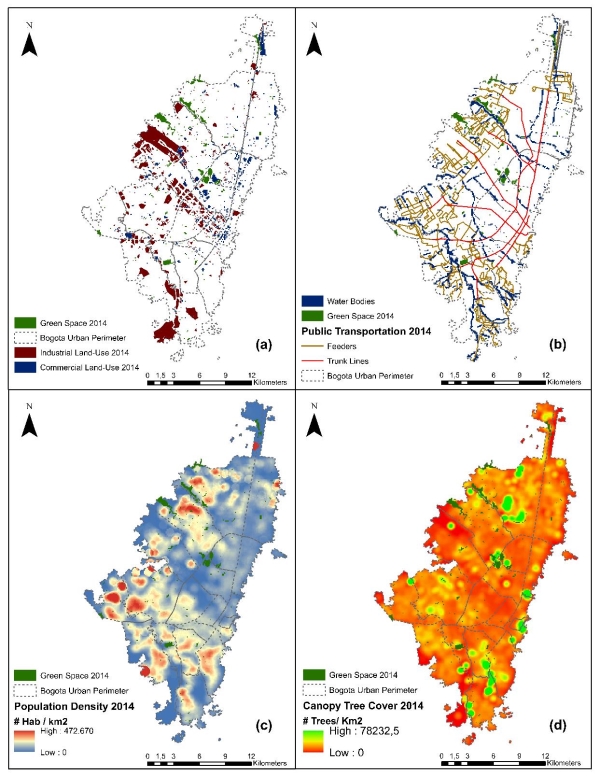
(a): Commercial and Industrial Land-use (b):
Public transportation network (c): Population Density (d): Canopy tree
cover
Figure 2. (Figure 1 continuation)
Finally, to be able to evaluate the interactions at the spatial
level, a Geographic Weighted Regression method was used. This method
allows evaluating spatial correlations between neighbouring cells,
increasing the specificity of the model and makes it more reliable, as
(Yang, Lu, Cherry, Liu, & Li, 2017) assures base on their experience
modelling the relationship between active mode travel demands and
ambient built-environment attributes; in which they checked that GWR has
higher prediction power and provides a more understanding of the spatial
variations in the relationships at local and global level. The GWR can
be represented by Equation 2:

Equation 2. GWR regression model representation
Where 𝑦𝑖 correspond to the GS per capita or dependable variable,
over time; 𝛽0(𝑢0𝑣0) correspond to the intercept of the model with
spatial coordinates, 𝛽𝑛(𝑢𝑛𝑣𝑛) represent the coefficient of each
independent variables in which values denotes the spatial coordinates
for each observation, same as, 𝑋𝑖 till 𝑋𝑛 which is the dimensional
vector of K independent variable over time; meanwhile the 𝜀 correspond
to the disturbance of the independent and identical distribution.
4. RESULTS
It is imperative to understand that significant changes occurred for
a period of only 10 years (Figure 2 and Figure 4) which could suggest
that within the results there will be interesting changes and
variations. An evaluation of the six selected variables, see Table 1,
for 2005 and 2014 were carried out. Firstly, these variables were
analysed to determine the relationship with GS per capita. With each of
these variables, an exploratory regression analysis was executed. These
consisted of a global combination of all variables and with it obtaining
the most suitable and descriptive model. Each of the six variables had
to meet the following criteria:
- Probability and Robust Probability (P-Value): Indicates a
coefficient is statistically significant (P<0.05).
- Variance Inflation Factor (VIF): Large values (VIF >7.5)
indicates redundancy among explanatory variables.
- R-squared and Akaike’s Information criteria (IACc): Measures of
model fit/performance.
- Join F and Wald Statistics: Indicates overall model significance
(p<0.05)
- Koener (BP) statistic: When this test is statistically
significant (p<0.05) the relationship modelled are not consistent.
- Jarque-Bera Statistic: When this test is statistically
significant (p > 0.05) the residuals are not normally distributed.
In addition, it was observed that only four of the six variables in
Table 1 were significant in the spatial regression model. Afterwards, an
OLS regression model was run with the most representative variables in a
global model. Results can be observed in Table 2 and Table 3. In these,
the only variable that is representative and can describe the GS per
capita in 2005 is population density; meanwhile, in 2014, it was added
the coverage of the public transport network as another representative
variable. This representability is mainly given by the p-value
statistics, even if the rest of the criteria was fulfilled. Likewise, it
is important to note that once the p-value is fulfilled, the
t-statistics will also be met.
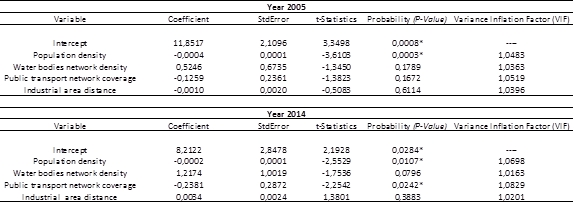
Table 2. Estimate OLS results for the
representative variables from 2005 & 2014

Table 3. Summary of descriptive statistics for
the OLS regression model
Once the results of the model have been obtained, it is observed that
at present there are only two variables that can explain the GS per
capita; of all the possible ones suggested by the literature.
This result suggests that Bogotá is a city that not only lacks green
areas for the benefit of its inhabitants but, there is no pattern in the
spatial distribution of these zones or enough variables to explain it,
something commonly found in cities in developing countries. However,
within the research, this was seen as an opportunity to construct a
scenario that allowed to explain the spatial distribution of the GS and
to quantify it at the level of cell resolution. That is why a much
greener scenario for the year 2040 was run; however, to avoid changing
any parameter in the “BoLD” model, a new run was decided in which only
the zoning will change based on the decree 06 of 1990, of the government
of Bogotá, in which establish on the articles 138 and 139 that in the
border of the river or the riparian zone of the river must be a
protection zone with more than 300 linear meters each side.
Figure 3 shows the modification in the zoning and the land-use map
for this new scenario. Figure 4 shows the new green zones, obtained
after processing the land-use map for 2040, together with the six
variables analysed for the previous years, all following the same
procedure described in the methods, with the exception of the canopy
tree cover.
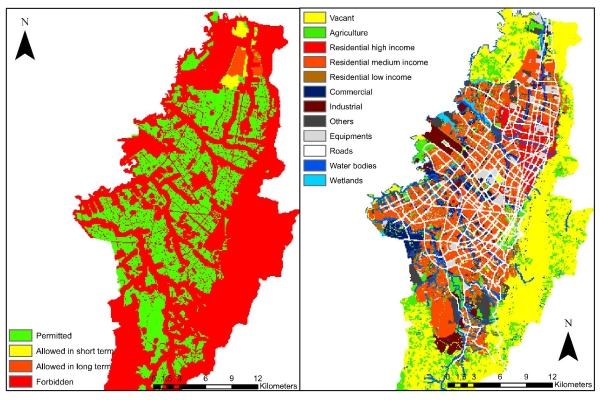
Figure 3. The new zoning and land-use map
obtained for this greener scenario in 2040
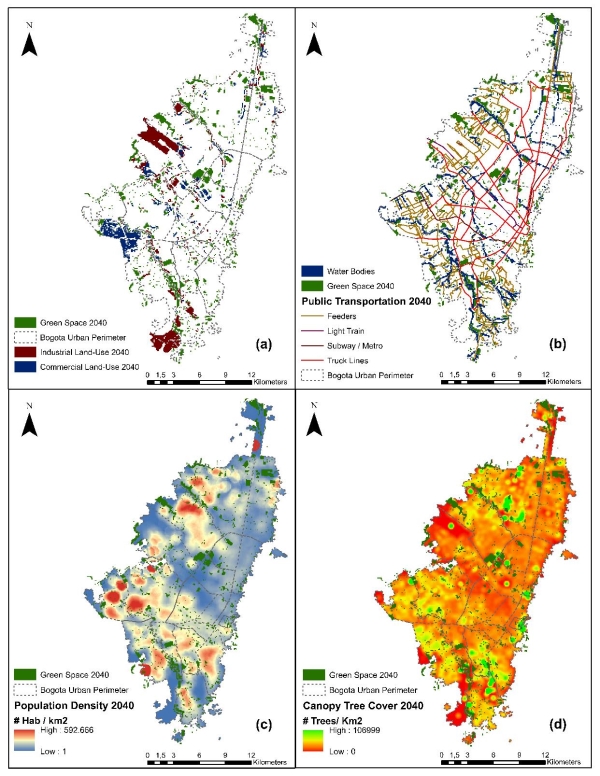
(a): Commercial and Industrial Land-use (b):
Public transportation network (c): Population Density (d): Canopy tree
cover
Figure 4. (Figure 1 continuation)
For this last variable the procedure that was carried out was the
following:
- Under the international literature, several cities aim to reach
an index of 0.30 - 0.50 canopy tree density per inhabitant (MIT,
2017)
- For the present study, it was considered that in 2040 Bogotá
would be at 0.25.
- By 2014 Bogota had 1,253,533 trees; considering the previous
indicator, in 2040 this would rise to 2,549,055 trees.
- However, growth would not be uniform. In 2014, 28 was the
average number of trees per square
Page 12 of 17 km; so in the future it was decided that all zones
above these value will represent 50% of the additional trees;
meanwhile, all the zones below the average, that it's almost all the
city, will be
the other half of the increase.
- So with this method, the average number of trees per km2 would
be 57 by 2040, as opposed to a
uniform extrapolation with an average of only 39 trees per km2. For
this scenery, an OLS regression model was run tested again in which
the expected result was a significant improvement compared to
previous years. The model was once again run with the previous six
variables. Once run it, four of the six variables met all the
criteria. Although it was a greener scenario, two variables were
unable to explain the location and distribution of the GS per
capita; they were: canopy tree coverture and the commercial distance
measured from the residential land use. In Table 4 and Table 5 it is
presented the general diagnosis of the OLS model with main four
representative variables.

Table 4. Estimate OLS results for the
representative variables for 2040

Table 5. Descriptive statistics for the OLS
regression model in 2040
With the results obtained within the OLS
regression model, it is observed that the global model meets
statistical significant. In the local model, the variables will
be evaluated at a cell resolution of 100m x 100m of residential
land use. The results of the GWR regression model can be
seen in Table 6 for each of the evaluated years. All the models
were evaluated with the four significant variables, in which it
was observed that at the local level the chosen variables have a
greater significance. Since the performance in the greener
scenario, the spatial pattern of these variables explains more
than the 47% of the GS per capita for the year 2040, as can be
seen in Figure 5 where the spatial distribution for the local R2
of the model is shown over the time.
|
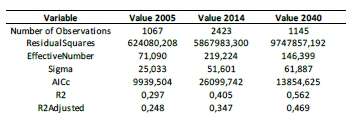
Table 6. GWR result for the three periods
of time
|
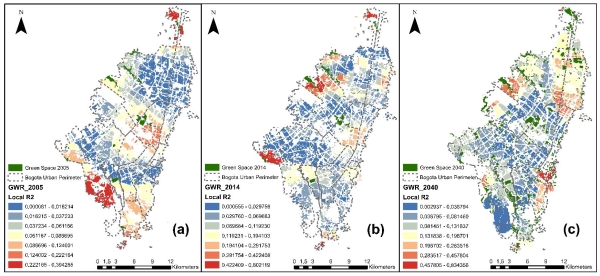
Figure 5. GWR Spatial distribution for the local
R2 for the UGGI per Capita the three periods of study (click
for larger size).
The significance of the model at global and local level, allows to
conclude that in the variables evaluated to quantify and measure GS per
capita, coincide with those observed in the literature; in turn, it is
observed that all the criteria for evaluating an indicator are met,
since they are measurable and quantifiable over time. It should be noted
that in the current context only two of the four variables can explain
the distribution of the current GS; however, under a scenario where the
normativity to protect ecosystems and UGS is enforced, the dynamics can
change. This is reflected in the fact that in a much greener scenario,
all the selected variables are statistically significant and explain in
a considerable way the spatial distribution of the green areas; which
allows to infer, that under the equations above, these variables can
establish at any resolution how much GS per capita there will be in
every residential cell in the future.
5. DISCUSSION
In Bogota since 2005, a master plan for the effective public space
was established, in which goals were set for 2019. These goals
stipulated that by 2015 Bogotá would have a per-capita public space of 6
m2. Today this value is only 4.10 m2. In turn, the same plan stipulated
that there would be continuous monitoring and updating of information on
indices and GS, as well as increasing and improving afforestation and
connectivity rates of urban ecosystems. All of the above would be done
with a view to protecting, preserving and guaranteeing the enjoyment of
these ecosystems for the community. However, Bogotá currently lacks
effective GS at the physical level, and there is no political interest
that generates clarity and continuity in this type of policy.
In this master plan of public space, there was no distinction made
between private, public, road medians and future GS. Also, it is not
clear in how must be interpreted the riparian zones near water bodies.
All of the above, generate a challenge in Bogotá when measure GS comes
forward; since the benefit and quantification for each of these spaces
is different from each other and should not be quantifiable as a whole.
Furthermore, the lack of up-to-date information, clear GS plans and
up-to-date information generates limitations within the micro-level
model.
This limitation occurs when running statistical models since the lack
of reliable and updated information will generate more uncertainty
within the model. Each variable considered has a unique temporal change
and spatial pattern. This implies that for each variable the past and
current state was known; however, it was necessary to make an estimate,
which is fundamental for land use change models and for decision makers.
In the case of transport infrastructure, it should be considered that
when looking at the area of influence of a transport network is not done
for the entire network but, specifically for the access points, since
they are in these where important changes occur; Likewise, for the case
of the variable of population density, it must be based on the fact that
the last official census that was carried out was in the year 2005. This
implies that the spatial distribution of the population could present
considerable changes compared to the year 2014, which would imply an
important bias for the distribution that would be presented in the year
2040; however, not considering this variable had omitted the most
representative and most important variable to measure the GS index in
the city. Also, it is important to remember that the other variables
that explain the model are mainly a product of the future land policies
and territorial planning, which can be changed and present over time.
Although the estimation of each of these variables may present some
weakness, calculating and predicting them are fundamental to validate
and simulate changes in a LUCC. The predictive models are based on
linear and spatial regressions, which allow us to evaluate their
representatives over time. In the case of an OLS regression, the
representability is determined not only by the p-value but also by the
specificity of the variables, which means, that exploratory variables
should describe the elasticity of the dependent variable. This indicates
the percentage of change of the phenomena due to the independent value
(e.g. an increase of population implies a reduction in the GS per
capita. Therefore the coefficient that is the numerical value of the
change must be negative), as shown in tables Table 2 and Table 4. Also,
it is important to always consider the standard deviation and the
residual errors as measurements of the quality of the model. In the end,
these indicate that the variables are consistent and the model is
correct.
GWR model allows evaluating the statistical and spatial impact of one
variable over another in a local context, or cell size. This tool allows
finding patterns such as areas where there is a greater correlation,
compared to areas where deviation and representability are negative.
This is a new way of analyzing and understanding the reasons why these
zones present this disjunction when explaining the phenomena. In the
case of the present study, if a Hot - Cold Spot analysis was made, it
would be observed that the areas with the lowest density of GS are those
with the lowest R2 and
lowest statistical representability, which generates a negative impact
when evaluating the model in a global way. In the opposite case, when
this raster analysis presents a high square R2,
it shows how explanatory the local model is and how there are incidence
and correlation between variables and model in general. Finally, the sum
of this allows bringing a global model to a local context, which can
change due to the resolution of the model and the information available.
Finally, the proposed scenario for 2040 showed that preserving of the
riparian zone, not only a greater GS was obtained, but the intervention
in the built area of the city was minimum. Also, considering the
population growth, not only an overall increase of the GS was achieved,
but in turn, it was observed that the standards of GS per capita in
urban Bogotá was above of the suggested by the WHO. It is important to
note that even when the “Bold” model includes seven neighbouring
municipalities, these were not considered due to the lack of available
information. The increase of green areas at the global level and the
index of GS per capita for each cell are observed in Table 7 and Figure
6, respectively.

Table 7. Global GS per capita for Bogotá
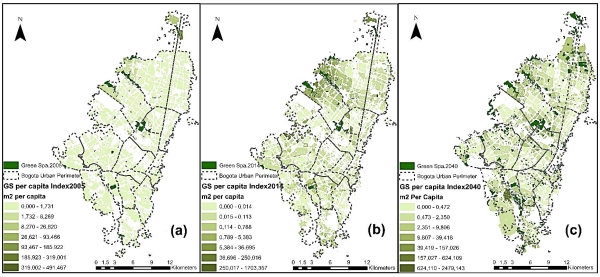
Figure 6 Change in UGS per capita over timey (click
for larger size).
6. CONCLUSIONS
The lack of GS in the past and present in the city of Bogotá shows a
problem that can still be reversed through clear and concrete actions.
Among these actions, it is crucial that the future city plan has a more
sustainable approach. That why the LUCC models are used, as it allows to
establish greener scenarios with the current city policy. Likewise, the
application of Local (GWR) and Global (OLS) regression models allows
proposing a methodology to quantify and measure the future GS per
capita, through present variables that are quantifiable and can be
measured over time. This methodology it is proposed as a way to measure
GS in CA models.
Within the research, it was taken into account not only the
international literature but also the national and case studies in
Bogotá; however, GS in Latin America and in, the Colombian case, are
very low; as this is not yet a priority for governments. It is also
important to highlight that there are variables that could be much more
representative, however such as housing price, crime rate, sustainable
projects, land value, urban biodiversity and more; but the lack of
updated and detailed information as well as the level of resolution
represent an important challenge when estimating future scenarios.
Finally, further research must be done in order to quantify the GS in
an integral way, since in compact cities the limiting of land is in
conflict with the preservation of ecosystems. That is why, in the long
term within these green areas should be counted new urban
infrastructures as green roofs, new urban developments and tree canopy
density as new GS, which can improve the quality of life of its
inhabitants and make cities much more green in an unconventional way.
7. ACKNOWLEDGMENTS
I would like to thank the guidance provided by Professor Daniel Paez
and the Group Sur. In turn, thank Professor Abbas Rajabifard and the
entire CSDILA team for all the technical support and literature
suggested for this research. Any opinions, findings and conclusions
expressed in this paper are those of the author and do not necessarily
reflect the views of the two research centres.
8. REFERENCES
Alcaldía de Medellín. (2011). BIO 2030 Plan Director Medellín,
Valle de Aburrá. Medellín: RMESA. Retrieved from
http://www.eafit.edu.co/centros/urbam/Documents/BOOKbio2030plandirectormedellin.pdf
Anderson, W., Guikema, S., Zaitchik, B., Pan, W., Pfeffermann, D.,
Clayton, D., & Emerson, R. (2014). Methods for Estimating population
density in Data-Limited areas: Evaluating Regression and Tree-Based
Models in Peru. PLos ONE. doi:10.1371/journal.pone.0100037
Anguluri, R., & Narayanam, P. (2017). Role of green space in urban
planning: Outlook towards smart cities. Urban Forestry & Urban
Greening, 25, 58 - 65.
Attwell, K. (2000). Urban land resource and urban planting - case
studies from Denmark. Landscape Urban Planning, 145 - 163.
Ayuntamiento de Barcelona. (2011). Barcelona green infrastructure
and biodiversity plan 2020. Barcelona: Hbitaturba. Retrieved from
file:///C:/Users/ja.franco953/Downloads/BCN2020_GreenInfraestructureBiodiversityPlan.pdf
Barbosa, O., Tratalos, J. A., Armsworth, P. R., Davies, R. G.,
Fuller, R. A., Johnson, P., & Gaston, K. J. (2007). Who benefits from
access to green space? A case study from Sheffield, UK. Landscape
and Urban Planning, 187 - 195.
Bibri, S. E., & Krogstie, J. (2017). Smart, sustainable cities of the
future: An extensive interdisciplinary literature review.
Sustainable Cities and Society, 31, 183-212.
Bocarejo, J. P., Portilla, I., & Pardo, M. A. (2013). Impact of
Transmilenio on density, land use, and land value in Bogotá.
Research in Transportation Economics, 78-86.
Bolund, P., & Hunhammar, S. (1999). Ecosystem services in urban
areas. Ecol. Econ., 29, 293 - 301.
Bottalico, F., Chirici, G., Giannetti, F., De Marco, A., Nocentini,
S., Paoletti, E., . . . Travaglini, D. (2016). Air pollution removal by
green infraestructures and urban forests in the city of Florence.
Agriculture and Agricultural Science Procedia, 8, 243 - 251.
Castillo, G. (18 de December de 2013). Indicadores Ambientales de
espacio público en Bogotá. Barcelona: Universitat politécnica de
catalunya. Obtenido de
http://upcommons.upc.edu/bitstream/handle/2099.1/20822/Mem%C3%B2ria%20-%20Ginna%20Alexandra%20CASTILLO.pdf?sequence=1
Connop, S., Vandergert, P., Eisenberg, B., Collier, M. J., Nash, C.,
CLough, J., & Newport, D. (2016). Renaturing cities using a
regionally-focused biodiversity-led multifunctional beneftis approach to
urban green infraestructure. Environmental Sicence & Policy.
doi:10.1016/j.envsci.2016.01.013
de la Barrera, F., Reyes-Paecke, S., & Banzhaf, E. (2016).
Indicators for green spaces in contrasting urban settings. Ecological
Indicators, 62, 212 - 219. doi:10.1016/j.ecolind.2015.10.027
Demuzere, M., Orru, K., Heidrich, O., Olazabal, E., Geneletti, D.,
Orru, H., . . . Faehnle, M. (2014). Mitigatin and adapting to climate
change: Multi-functional and multi-scale assessment of green urban
infraestructure. Journal of Environmental Management, 146, 107
- 115.
Duh, J. D., Shandas, V., Chang, H., & George, L. A. (2008). Rates of
urbanisation and the resiliency of air and water quality. Science of
the Total Environment, 400, 238 - 256.
Fan, P., Xu, L., Yue, W., & Chen, J. (2016). Accessibility of public
urban green space in an urban periphery: The case of Shangai.
Landscape and Urban Planning. doi:10.1016/j.landurbplan.2016.11.007
Force, U. G. (2002). Better Places–Final report of the Urban Green
Spaces Task Force. London: Green Spaces. Obtenido de
http://www.ocs.polito.it/biblioteca/verde/taskforce/gspaces_.pdf
Gedge, D. (2015, 10 26). 1 European Urban Green Infrastructure
conference 2015. Retrieved from Urban Green Infrastructure:
http://urbangreeninfrastructure.org/green-tramways-transport/
Gupta, K., Kumar, P., Pathan, S. K., & Sharma, K. P. (2012). Urban
Neighborhood Green Index - A measure of Green spaces in urban areas.
Landscape and Urban Planning, 105, 325 - 335.
doi:10.1016/j.landurbplan.2012.01.003
Gupta, K., Roy, A., Luthra, K., Maithani, S., & Mahavir. (2016).
GIS-based analysis for assessing the accessibility at hierarchical
levels of urban green spaces. Urban Forestry & Urban Greening,
18, 198 - 211. doi:10.1016/j.ufug.2016.06.005
He, C., Okada, N., Zhang, Q., Shi, P., & Li, J. (2008). Modelling
dynamic urban expansion processes incorporating a potential model with
cellular automata. Landscape and Urban Planning, 86, 79 - 91.
doi:10.1016/j.landurbplan.2007.12.010
He, C., Zhang, Q., Shi, P., Okada, N., & Zhang, J. (2006). Modeling
urban expansion scenarios by coupling cellular automata model and system
dynamic model in Beijing, China. Applied Geography, 323 - 345.
He, J., Li, C., Yu, Y., Liu, Y., & Huang, J. (2017). Measuring urban
spatial interaction in Wuhan Urban Agglomeration, Central China: A
spatially explicit approach. Sustainable Cities and Society, 32,
569 - 583. doi:10.1016/j.scs.2017.04.014
James, P., Magee, L., Scerri, A., & Steger, M. B. (2015). Urban
Sustainability in Theory and Practice: Circles of Sustainability.
London.
Jim, C. Y. (2004). Green-space preservation and allocation for
sustainable greening of compact cities. Cities, 21(4), 311 -
320. doi:10.1016/j.cities.2004.04.004
Jim, C. Y., & Chen, S. S. (2003). Comprehensive greenspace planning
based on landscape ecology principles in compact Nanjing city, China.
Landscape and Urban Planning, 65, 95 - 116.
Jim, C. Y., & Chen, W. Y. (2006). Impacts of urban environmental
elements on residential housing prices in Guangzhou (China).
Landscape and Urban Planning, 78, 422 - 434.
Jo, H. K. (2002). Impacts of urban greenspace on offsetting carbon
emissions for middle Korea. Journal of Environment, 65, 95 -
116.
MIT. (2017). Treepedia: Exploring the Green Canopy in cities
around the world. Boston: MIT. Obtenido de
http://senseable.mit.edu/treepedia
Natural England. (2010). "Nature Nearby" - Accessible Natural
Greenspace Guidance. London: Natural England. Obtenido de
www.naturalengland.org.uk/docunents/other/nature_nearby.pdf
New Yorkers for Parks. (2010). The Open Space Index. New York:
New Yorkers for Parks. Obtenido de
http://www.ny4p.org/research/osi/LES.pdf
Norton, B. A., Coutts, A. M., Livesley, S. J., Harris, R. J., Hunter,
A. M., & Williams, N. S. (2015). Planning for cooler cities: A framework
to prioritise green infrastructure to mitigate high temperatures in
urban landscapes. Landscape and Urban Planning, 134, 127 - 138.
Paez, D. E., & Escobar, F. (2016). Urban transportation scenarios in
a LUCC model: a case study in Bogota, Colombia. Bogotá.
Pakzad, P., & Osmond, P. (2016). Developing a sustainability
indicator set for measuring green infrastructure performance. Social
and Behavioral Sciences, 68 - 79.
Pauleit, S., & Duhme, F. (2000). Assessing the environmental
performance of land cover types for urban planning. Landscape Urban
Planning, 52, 1 - 20.
Scopellieti, M., Carrus, G., Adinolfi, C., Suarez, G., Colangelo, G.,
Lafortezza, R., . . . Sanesi, G. (2016). Staying in touch with nature
and well-being in different income groups: The experience of urban parks
in Bogotá. Landscape and Urban Planning, 148, 139 - 148.
Tzoulas, K., Korpela, K., Venn, S., Yli-Pelkonen, V., Kazmierczak,
A., Niemela, J., & James, P. (2007). Promoting ecosystem and Human
health in urban areas using Green Infrastructure: A literature review.
Landscape and Urban Planning, 81, 167 - 178.
WHO. (s.f.). Urban Green Spaces and Health: A review of the evidence.
WHO Regional Office for Europe. Copenhagen: WHO. Obtenido de
http://www.euro.who.int/__data/assets/pdf_file/0005/321971/Urban-green-spaces-and-health-review-evidence.pdf?ua=1
Wolch, J. R., Byrne, J., & Newell, J. P. (2014). Urban green space
public health, and Environmental Justice: The challenge of making cities
"Just green enough". Landscape and Urban Planning, 125, 234 -
244. doi:10.1016/j.landurbplan.2014.01.017
Wu, J. (2014). Urban ecology and sustainability: The state of the
science and future directions. Landscape and Urban Planning,
209-221.
Yang, H., Lu, X., Cherry, C., Liu, X., & Li, Y. (2017). Spatial
variations in active mode trip volume at intersections: a local analysis
using geographically weighted regression. Journal of Transport
Geography, 64, 184 - 194. doi:10.1016/j.jtrangeo.2017.09.007
Yin, X., Olesen, J. E., Wang, M., Ozturk, I., & Zhang, H. (2016).
Impacts and Adaptation of the cropping systems to climate change in the
northeast Farming Region of China. European Journal of Agronomy, 78,
60-72.
Zeng, C., Zhang, M., Cui, J., & He, S. (2015). Monitoring and
modelling urban expansion - A spatially explicit and multi-scale
perspective. Cities, 92-103. doi:10.1016/j.cities.2014.11.009
Zuccalà, M., & Verga, E. S. (2017). Enabling energy smart cities
through urban sharing ecosystems. Energy Procedia, 826 - 835.
|