A Quantitative Geo-Evaluation of Crowdsourcing and
Wisdom of the Crowd 1)
Mapping and Geo-information Engineering
Technion - Israel Institute of Technology
Haifa, Israel
1) This paper was presented
at the commission 3 meeting, 4-7 November 2014 in Bologna, Italy. The
paper aims at distinguishing between the Crowdsourcing and Wisdom of the
Crowd, via the quantitative and theoretical examination of two widely
used location based services: OpenStreetMap (OSM), and Waze.
ABSTRACT
The revolution of web 2.0 has brought the development of two
important working methodologies: Crowdsourcing and Wisdom of the Crowd.
The two are widely used today in a variety of research and working
fields, let alone within the mapping and geo-information discipline.
Still, these two terms are commonly misused and replaced. This paper
aims at distinguishing between the two, via the quantitative and
theoretical examination of two widely used location based services:
OpenStreetMap (OSM), and Waze. Four indices are defined and examined
within the scope of this research, aiming to investigate and emphasize
on the differences existing between the two terms in respect to these
services, namely: diversity, decentralization, independency, and
aggregation. It was found that OSM is a very good example to a
crowdsourcing project and Waze is more wisdom of the crowd project than
crowdsourcing project.
1. INTRODUCTION
Since the 1990s, there has been significant development of online
publishing tools, and particularly of the World Wide Web (WWW)
(Berners-Lee et al., 1992). Such developments have simplified
interaction between users and navigation through enormous amounts of
data and information. The invention of the WWW is especially meaningful,
mainly due to the development of its interface (Bowman et al., 1994),
which enabled the visualization of geographic information. Years later,
new mapping applications deluged the Internet; this trend became known
as 'The Geographic World Wide Web' (or 'the GeoWeb') (Haklay et al.,
2008).
The GeoWeb became a platform for the breakthrough of online
Geographical Information Systems (GIS) in the mid 2000's. This made it
possible for the mapping field to become not only an experts' domain,
but also a public domain. Users all over the world were involved in data
processing, mainly thanks to Web 2.0 technologies, thus public mapping
has become widely used (Haklay, 2010). More and more mapping and
location based projects and services were using group of volunteers to
collect and disseminate data (as opposed to authoritative mapping
agencies), making it possible to create and update geospatial
information infrastructure, such as an online map, having the aspiration
to actually replace licensed surveyors, cartographers and geographer
experts, e.g., authoritative sources. This phenomenon is known as
neogeography, and has contributed to the development of two important
working methodologies: Crowdsourcing and Wisdom of the Crowd, widely
used today within the mapping and geo-information discipline.
1.1 Wisdom of the Crowd
The expression Wisdom of the Crowd was coined by Surowiecki (2004),
claiming that "Large groups of people are smarter than an elite few, no
matter how brilliant – better at solving problems, fostering innovation,
coming to wise decisions, even predicting the future." The author
describes that the crowd can be any group of people that "can act
collectively to make decisions and solve problems". According to the
author, big organizations, such as a company or a government agency,
small groups like a team of students, and groups that are not really
aware of themselves as groups, such as gamblers, may act as a crowd.
However, to make a ‘wise’ crowd, four main characteristics are required:
- Diversity – each individual contributes different pieces of
information.
- Decentralization – the crowd answers are not influenced from the
hierarchy above them (e.g., founder or funder).
- Independence – a person's opinion is not affected by people in
his close vicinity but only from his or her own opinion.
- Aggregation – a mechanism that unifies all individual and
independent opinions into a collective decision or deduction.
1.2 Crowdsourcing
Crowdsourcing has received a considerable attention over the past
decade in a variety of research fields, such as economics, funding,
computing, mapping etc., also among companies, non-profit organizations
and academic communities (Zhao and Zhu, 2014).
The term Crowdsourcing was coined by Howe (2006a), and since then is
increasingly being expanding. Howe's definitions refer to a new business
model that expanded due to web innovations (Brabham, 2008). The two
preferred definitions by Howe are:
- "The White Paper Version: Crowdsourcing is the act of taking a
job traditionally performed by a designated agent (usually an
employee) and outsourcing it to an undefined, generally large group
of people in the form of an open call."
- "The Soundbyte Version: The application of open-source
principles to fields outside of software."
The Merriam-Webster online dictionary defines Crowdsourcing as "The
practice of obtaining needed services, ideas, or content by soliciting
contributions from a large group of people and especially from the
online community rather than from traditional employees or suppliers."
The most cited article dealing with the term Crowdsourcing is Brabham
(2008), describing the term as "a distributed problem-solving model, is
not, however, open-source practice. Problems solved and products
designed by the crowd become the property of companies, who turn large
profits off from this crowd labor. And the crowd knows this going in".
Moreover, the author claims that "Crowdsourcing can be explained through
a theory of crowd wisdom, an exercise of collective intelligence, but we
should remain critical of the model for what it might do to people and
how it may reinstitute long-standing mechanisms of oppression through
new discourses… It is a model capable of aggregating talent, leveraging
ingenuity… Crowdsourcing is enabled only through the technology of the
web".
Estelles-Arolas and Gonzalez-Ladron-de-Guevara (2012) tried to embed
an integrated definition to Crowdsourcing: "Crowdsourcing is a type of
participative online activity in which an individual, an institution, a
non-profit organization, or company proposes to a group of individuals
of varying knowledge, heterogeneity, and number, via a flexible open
call, the voluntary undertaking of a task. The undertaking of the task,
of variable complexity and modularity, and in which the crowd should
participate bringing their work, money, knowledge and/or experience,
always entails mutual benefit. The user will receive the satisfaction of
a given type of need, be it economic, social recognition, self-esteem,
or the development of individual skills, while the crowdsourcer will
obtain and utilize to their advantage that what the user has brought to
the venture, whose form will depend on the type of activity undertaken."
1.3 Aim of Paper
Wisdom of the Crowd is commonly confused with Crowdsourcing. For
example, does Wikipedia have a nature of the Wisdom of the Crowd or
Crowdsourcing? Wu et al. (2011) claimed that Wikipedia is the Wisdom of
the Crowd; however, Howe (2006b) and Huberman et al. (2009) claimed that
it is a Crowdsourcing paradigm. Moreover, there exist research that does
not distinguish between the two terms (e.g., Vukovic, 2009; Stranders et
al., 2011); such a distinction should be specified.
Aforementioned characterizations of Crowdsourcing and the Wisdom of
the Crowd result from an analysis related to a wide diversity of fields
and disciplines. However, there are no clear definitions to both terms
within the geospatial domain, e.g., services, applications and
processes. The aim of this research paper is to analyze these terms and
working paradigms with respect to social geospatial and location based
services, emphasizing on special and unique attributes and
characterizations related to mapping and geo-information. Special effort
is given to try and find the differences between the two terms, with the
use and analysis of two key social location based services used by tens
of millions of users around the globe: OSM (© OpenStreetMap
contributors) and Waze (© 2009-2014 Waze Mobile).
This paper is structured as follows: section 2 provides with a review
of state-of-the-art and relevant research papers, followed by section 3
describing the methodology for choosing the indices (characteristics) to
facilitate the examination of the two social location based services,
with a general introduction of the two. Section 4 analyzes the four
indices in respect to the two services to provide a clear identification
to the two terms and paradigms. The results of the analysis are
presented in section 5, while section 6 concludes the article.
2. RELATED WORK
Many researchers investigate and examine the term Crowdsourcing
within the scope of its implementation. A review of the term is made in
Hudson-Smith et al. (2009), which describes Crowdsourcing by using
principles, concepts and ideas of the term Wisdom of the Crowd.
Following the authors examples to new approaches of collecting, mapping
and sharing geocoded data, and definition given in the article to
Crowdsourcing, it is made clear that they see little difference (if at
all) between the two terms. Moreover, the authors analyze the
neogeography definition through online mapping tools, such as
GMapCreator and MapTube.
Bihr (2010), carrying out a comparison between the two terms,
describes the general similarities, as well as the differences, that
exist between the two, while giving several examples. Perhaps one of
author’s more significant claims is that …"Crowdsourcing can enable the
Wisdom of the Crowd (but does not have to)"; still, this is not
mandatory.
In Alonso and Lease (2011), Crowdsourcing is explained through the
term Wisdom of the Crowd. In addition, the authors present examples of
the concept such as Mechanical Turk, Crowdflower etc., introduce the
motivation for volunteers to contribute, and explain advantages and
disadvantages of using the crowd.
Recent research tries to emphasize on finding clear and consistent
definitions to the term Crowdsourcing. However, it is clear, and to some
extent surprising, that there is no single definition of Crowdsourcing,
despite the many attempts searching for such a definition. Schenk and
Guittard (2011) compare the term Crowdsourcing with several similar
concepts (such as: Open Innovation, User Innovation and Free-Libre-Open
Source Software), highlighting existing dissimilarities. In addition,
the authors focus on defining typology of Crowdsourcing from two
different views: 1) the integration of the crowd information, and, 2)
the selection of one answer among provided crowd information. Tasks that
can be crowdsourced were introduced and divided into three main groups:
simple tasks (e.g. data collection), complex tasks (e.g. problem
solving), and creative tasks (e.g. design). Finally, benefits (such as
cost, quality, motivations and incentives), and drawbacks (such as lack
of contributors, request definition, etc.) of Crowdsourcing were
presented.
In Estelles-Arolas and Gonzalez-Ladron-de-Guevara (2012), an
integrated crowdsourcing definition is given, where authors try to find
a wide definition that will cover as many Crowdsourcing processes as
possible (see definition in Section 1.2). The author’s definition is a
result of analyzing 40 original definitions, and consists of eight
characteristics, as follows: 1) the defined crowd, 2) the task with
clear goal, 3) clear recompense obtained, 4) identified crowdsourcer, 5)
defined compensation (by crowdsourcer), 6) the type of process, 7) the
call to participate, and, 8) the medium usage. These characteristics
were analyzed through eleven known projects, such as Wikipedia, YouTube
– and more. According to the characteristics, the authors concluded that
Wikipedia and YouTube, for example, are ambiguous when it comes to a
clear Crowdsourcing definition. That is because characteristics number
4, 5 and 7 do not exist in Wikipedia, while in YouTube, only
characteristics 1 and 8 exist.
Zhao and Zhu (2014) made an overview of the current status of
Crowdsourcing research, trying to present a critical examination of the
visible and invisible substrate of Crowdsourcing research, and pointed
on possible future research directions. Moreover, the paper
distinguished between Crowdsourcing and three related terms: Open
Innovation, Outsourcing and Open Source. In addition, the authors
presented a conceptualization framework of Crowdsourcing that is based
on four questions: 1) who is performing the task, 2) why are they doing
it, 3) how is the task performed, and, 4) what about the ownership and
what is being accomplished?
Summarizing the above, it is clear that although the term
Crowdsourcing does not have a comprehensive definition, the term Wisdom
of the Crowd has a clear definition, as presented earlier in section
1.1. Still, no up-to-date article was found that tried to analyze the
term Wisdom of the Crowd with respect to new projects. Moreover, no
research was found that tried to define these two terms specifically in
respect to the geospatial scientific discipline and geo-services, which
is the aim of this paper.
3. METHODOLOGY
Crowdsourcing and Wisdom of the Crowd are often terminologically
intertwined and indefinite. This is probably because the use of these
terms is common and widespread in diversity of fields and disciplines –
which are also very dynamic and changing, or because they are still not
enough established, and continue to adapt and transform. The comparison
between the two terms is demonstrated in respect to two popular social
location based services and applications that incorporate processes
having geographic and geospatial characterization: OSM and Waze. The two
have tens of millions of users worldwide. They offer location based
services, in which volunteers are the fundamental core of creating the
services via the data they collect and share.
A review of abovementioned articles and an examination of uses and
definitions that appear in this context have led to the selection of the
following four characteristics, or indices, which characterize various
processes involved in the services analyzed: 1) Diversity, 2)
Decentralization, 3) Independency, and, 4) Aggregation. A comprehensive
explanation of these indices is given in the next section.
An overview of the two social location based services is necessary to
understand the background of the proposed analysis that is carried out
in section 4:
3.1 OSM
OSM is a collaborative online project and an open-source editable
vector map of the world, created and updated by volunteers. The project
aim is to create a map that is editable and free to use, especially in
countries where geographic information is expensive and unreachable for
individuals and small organizations, and also frequently changed (Haklay
et al., 2008) (Figure 1). As such, OSM is an alternative mapping service
in respect to other authoritative sources. Users can view and edit the
underlying OSM data, upload GPX files (GPS traces) from hand-held GPS
units or correct errors in local areas according to satellite imagery
and out-of-copyright maps, which are integrated into the mapping
interface (Haklay and Weber, 2008) (Figure 2). OSM is constantly widens
worldwide, and nowadays match other mapping services, such as the
commercial Google Maps, due to the increase of qualitative aspects of
OSM, such as accuracy, completeness and reliability.
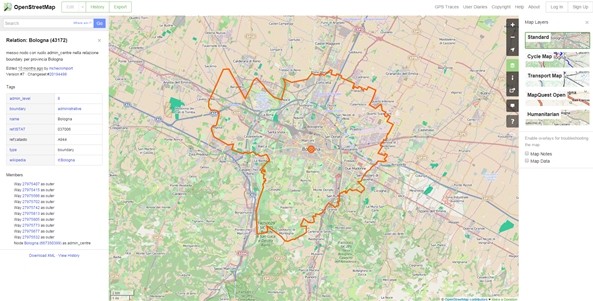
Figure 1. An example of an OSM map and viewing interface – Bologna,
Italy (source: OpenStreetMap.com).
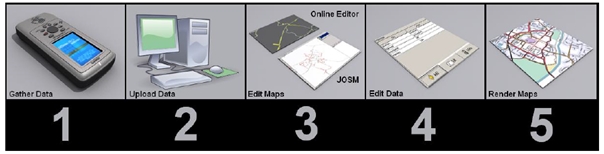
Figure 2. Schematics workflow for creating OSM maps (source: Haklay and
Budhathoki, 2010).
3.2 Waze
Waze is a social community-and-GPS-based traffic and geographical
navigation service. Drivers living and driving in the same area can
share real-time traffic and road information with others. Data is
collected automatically from the driver simply by driving with an open
Waze app, and is based on the car direction, location and speed, all
sent to Waze servers for further analysis and dissemination of service
to other users (Figure 3). Users can actively report traffic jams,
accidents, road dangers, fuel stations with the lowest gas price along
the route, speed and police control, and hazards on the road, etc.
Moreover, from the online map editor users can add new roads or update
existing ones, add landmarks, house numbers, etc. The collected data are
aggregated and provided to the community as alerts, traffic flow updates
– and more (Figure 4).
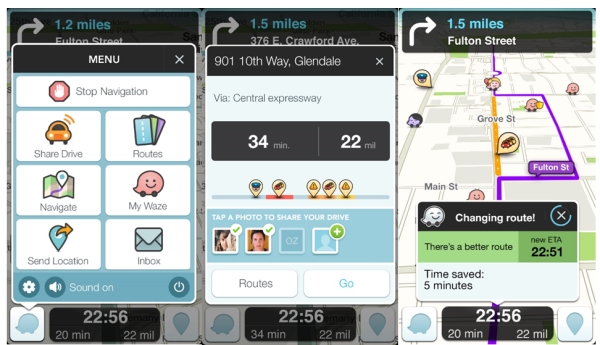
Figure 3. Waze interface (left to right): 1) Main menu screen; 2)
Estimated time of arrival (ETA) screen and route option; 3) ETA update
screen due to live update traffic (source: Waze.com).
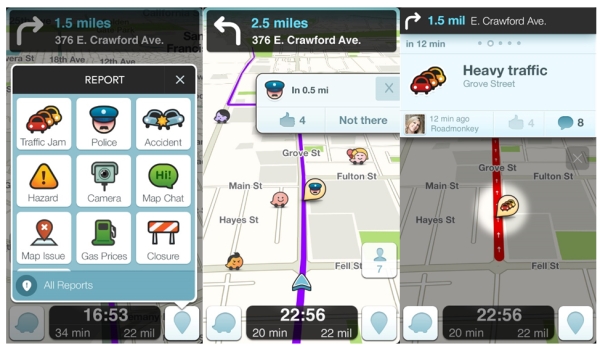
Figure 4. Waze interface (left to right): 1) Report Menu screen; 2)
Hazard alert screen; 3) Traffic jam report screen (source: Waze.com).
4. IDENTIFICATION OF TERMS
4.1 Diversity
Volunteers participating in a task defined as Wisdom of the Crowd
must produce different – and diverse – pieces of information. In fact,
this is also the case for a task configured as Crowdsourcing, where the
volunteers should contribute diverse data. Diversity encourages a
variety of innovative ideas (Surowiecki, 2004), and in the mapping
discipline it helps to cover wide topographic areas while increasing the
certainty and update of the (already exiting) data.
OSM and Waze gained big success thanks to the wide variety of
geospatial information that volunteers contribute. In OSM, volunteers
can add buildings, roads, shops, schools and everything needed to
complete missing information. Waze users (drivers) can add new roads,
place of accidents, police traps, road dangers, or can map a gas station
with the lowest gas prices. According to current quality standards and
definitions in respect to crowdsourced volunteered geographical
information (e.g., Haklay, 2010), the existence of a wide range of
contributors for the two services, which exist in this category, should
improve the geospatial and geometric completeness of information,
together with the spatial as well as the temporal quality of the mapping
infrastructure. Therefore, this index is significant both in
Crowdsourcing and in Wisdom of the Crowd, and thus important to the
analysis of the two services chosen – but with different magnitudes.
4.2 Decentralization
Decentralization is strongly correlated to the diversity index, due
to the fact that similarity among the people having influence reduces
the variety of new products: "…the more similar the ideas they
appreciate will be, and so the set of new products and concepts the rest
of us see will be smaller than possible" (Surowiecki, 2004). Moreover,
decentralized organizations have the same aspect: "power does not fully
reside in one central location, and many of the important decisions are
made by individuals based on their own local and specific knowledge
rather than by an omniscient or farseeing planner" (Surowiecki, 2004).
Thus, the results derived from Wisdom of the Crowd will be more
innovative when they are decentralized. The Crowdsourcing tasks have the
same advantages of decentralized sources, i.e. funders or agents. Hence,
the two terms should have a relatively high rate of decentralization
aspects within services.
If commercial companies can gain certain influence on the data
collected, i.e., they can contribute data, such as gas station offering
the cheapest gas price, such that they can directly effect on the
driver's chosen route and deviate it (as with Waze). This might lead to
users not trusting the information – and consequently quality of service
- they receive and gain. Namely, services that are based on ‘the crowd’
aspire to get true and accurate information, and as such
decentralization helps to achieve this, especially in respect to a
centralized process. It is assumed that public organizations should
maintain objectivity, while private and commercial companies might be
biased in favor of their interests. While these concerns exist in
relation to major services and projects, it is assumed that it does not
occur here, due to the decentralization factor.
4.3 Independency
Independent answer is essential in Wisdom of the Crowd: "independence
of opinion is both a crucial ingredient in collectively wise decisions
and one of the hardest things to keep intact" (Surowiecki, 2004). In
Crowdsourcing, independent contribution (as in mapping) is important,
but still not a crucial aspect, since contributors can be affected by
contributions made by other contributors – though still having no effect
on the final product. Therefore, it can be seen that while independency
is essential in Wisdom of the Crowd, it is less crucial for
Crowdsourcing.
For example, if a volunteer sees that there is a good mapped area in
OSM, possibly he/she will search for an alternative less mapped area to
map. However, when a contributor is mapping a chosen area he/she should
map according to his own data and knowledge. Moreover, as in Waze, the
application map needs independent data - all drivers should supply their
own driving route, and report their own alerts. On the other hand, a
driver's route is influenced from all the information gathered from
other drivers, thus having a sort of a 'chicken or an egg' effect.
4.4 Aggregation
In Crowdsourcing, the volunteers serve as sensors (especially in
mapping projects) to provide the needed data (Goodchild, 2007). There is
no aggregation during the process. However, Wisdom of the Crowd takes
place only if an aggregation process is implemented on the volunteers'
contribution. "If that same group, though, has a means of aggregating
all those different opinions, the group's collective solution may well
be smarter than even the smartest person's solution" (Surowiecki, 2004).
In OSM, the environment is mapped by users, whereas the most current
update is added to OSM and considered as the final version. Thus, there
is no aggregation measure when an OSM map is produced. However, in Waze,
to receive accurate information about the place and time of a traffic
jam, an aggregation of all drivers' ’reports’ is essential and crucial –
without this, the Waze service will not exist. Hence, aggregation is one
of the most prominent indices that defer between the two terms, i.e.,
Wisdom of the Crowd must have an aggregation measure while Crowdsourcing
does not. Moreover, the service of Waze is expanding thanks to the
aggregation of their users' updates, hence 'going social'.
5. ASSESSMENT AND RESULT ANALYSIS
According to the analysis and explanation given in the previous
section, a system of score (on the scale of 1-10) is given to each
service, in respect to the four indices. High score represents the
necessity of the index in the service; respectively, low score means low
necessity of the index (Table 1).
Table 1: Indices score to services: columns represent the two
services, and rows represent the four indices. Score is on a 1-10 scale,
where 1 represents the lowest influence of the index on the service, and
10 the highest influence.
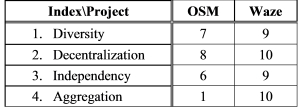
A weight system is given to each index in respect to the two terms –
how significant or influential the index is to the term. We have
analyzed each index in respect to both terms, deciding on the most
appropriate score (same scale as in Table 1): Crowdsourcing and Wisdom
of the Crowd (Table 2).
Table 2: Indices score to terms: columns represent the two terms, and
rows represent the four indices. Score is on a 1-10 scale, as
abovementioned.
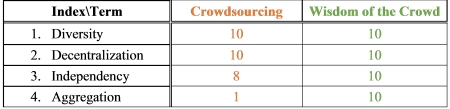
Finally, a formula was modeled to help and define the two services
either as Crowdsourcing or as Wisdom of the Crowd. This is done by
implementing two steps:
- Dividing the score of Table 1 by the score of Table 2, thus
obtaining a normalized score for the index.
- Calculating the average and the Standard Deviation (SD) of each
column, i.e., service, depicted in Table 3.
Score with value of 1 means that a service correlates absolutely to
the analyzed term (either Crowdsourcing or Wisdom of the Crowd, column
left (orange) and right (green), respectively). Score with a value that
is lower than 1 or more than 1 means that a service does not reflect in
full either of the terms; the farthest the value from 1 is – the less
correlation exists to the term.
Examining Table 3, it is clear that the average score of OSM is close
to 1 for Crowdsourcing (0.81), and much smaller than 1 for Wisdom of the
Crowd (0.55), also having a very small SD value (0.13) for
Crowdsourcing. This means that OSM has very good correlation to having
the characteristics of a Crowdsourcing service. Waze, on the other hand,
correlates almost perfectly as a Wisdom of the Crowd service, having a
0.95 score with a very small SD value (0.06). The Waze score of being a
Crowdsourcing service is very high (3.26) with a high SD value (4.50),
meaning that it cannot be characterized as a Crowdsourcing service.
Table 3: Each service has two normalized scores: Crowdsourcing (left,
orange), and Wisdom of the Crowd (right, green). Score of 1 represents
absolute correlation.
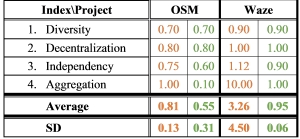
6. CONCLUSIONS
This research aimed at developing a quantitative measure to
distinguish between the terms Crowdsourcing and Wisdom of the Crowd with
respect to social location based services, which are geospatial by
nature. Since both paradigms are tightly interrelated and do not have a
clear definition – mainly when location based services are at hand – a
new measuring analysis system was needed, and hence developed for this
research paper. A system of four indices was decided upon, in which two
key services where analyzed in respect to the four indices. Analysis
showed that OSM is strongly correlated as a Crowdsourcing service (or
project), as it was assumed. In contrast, Waze showed the
characteristics of Wisdom of the Crowd service, and as such was more
correlated to this working paradigm, mostly because its core service is
based on an aggregation process; without this, such service could not
exist, and hence could not serve with the adequate and expected service.
Moreover, the analysis showed that a process having a Crowdsourcing
nature could be transformed to be a Wisdom of the Crowd one. This occurs
when volunteers continue updating data, while an appropriate aggregation
measure is established. However, when the volunteers' answers and
solutions are collected, and only one or a relatively small number are
chosen, this has a resemblance of a Crowdsourcing service, since an
aggregation process is not done. Further experiments with other indices
and services can serve with a better quantitative clarification of the
two terms, and the related processes they encompass. Still, due to rapid
technological developments and services available, such a clear
definition might be hard to achieve, since it seems that both terms are
in principle flexible and dynamic. Moreover, the services themselves
might not conform to the terms rubrics and characteristics, since they
themselves continue to evolve, adding continuously new features and
attributes.
REFERENCES
- Alonso, O. and Lease, M., 2011. Crowdsourcing 101: Putting the
WSDM of Crowds to Work for You. In: Proceedings of the Fourth ACM
International Conference on Web Search and Data Mining (WSDM), pp.
1-2, Hong Kong, China. Slides available online at
http://ir.ischool.utexas.edu/wsdm2011_tutorial.pdf
- Berners-Lee, T., Cailliau, R., Groff, J. and Pollermann, B.,
1992. World-Wide Web: The Information Universe. Internet Research,
vol. 2, no. 1, pp. 52-58.
- Bihr, P., 2010. Crowdsourcing 101: Crowdsourcing vs Wisdom of
the Crowd. Slides available online at
http://www.slideshare.net/thewavingcat/101-crowdsourcing-vs-wisdom-of-the-crowd
(accessed on: 2/10/2014).
- Bowman, C.M., Danzig, P.B., Hardy, D.R., Manber, U. and
Schwartz, M.F., 1994. Harvest: A Scalable, Customizable Discovery
and Access System. Technical rept. Colorado Univ. at Boulder Dept of
Computer Science.
- Brabham, D.C., 2008. Crowdsourcing as a Model for Problem
Solving: An Introduction and Cases. Convergence: The International
Journal of Research into New Media Technologies, vol. 14, no. 1, pp.
75-90.
- Estelles-Arolas, E. and Gonzalez-Ladron-de-Guevara, F., 2012.
Towards an integrated crowdsourcing definition. Journal of
Information Science, vol. 38, no. 2, pp. 189-200.
- Goodchild, M., 2007. Citizens as sensors: the world of
volunteered geography. GeoJournal, vol. 69, no. 4, pp. 211-221.
- Haklay, M., 2010. How good is volunteered geographical
information? A comparative study of OpenStreetMap and ordnance
survey datasets. Environment and Planning B: Planning & Design, vol.
37, no. 4, pp. 682-703.
- Haklay, M. and Weber P., 2008. OpenStreetMap: User-Generated
Street Maps, IEEE Pervasive Computing, vol. 7, no. 4, pp. 12-18.
- Haklay, M. and Budhathoki, N.R., 2010. OpenStreetMap – Overview
and Motivational Factors. Horizon Infrastructure Challenge Theme
Day. University of Nottingham, UK.
- Haklay, M., Singleton, A. and Parker, C., 2008. Web Mapping 2.0:
The Neogeography of the GeoWeb. Geography Compass, vol. 2, no. 6,
pp. 2011–2039.
- Howe, J., 2006a. Crowdsourcing: A Definition (weblog, 2 June),
URL (accessed 2 July 2014):
http://crowdsourcing.typepad.com/cs/2006/06/crowdsourcing_a.html
- Howe, J., 2006b. The Rise of Crowdsourcing. Wired Magazine, vol.
6, no. 14, pp. 183.
- Huberman, B.A., Romero, D.M. and Wu, F., 2009. Crowdsourcing,
attention and productivity. Journal of Information Science, vol. 35,
no. 6, pp. 758-765.
- Hudson-Smith, A., Batty, M., Crooks, A. and Milton, R., 2009.
Mapping for the Masses: Accessing Web 2.0 Through Crowdsourcing.
Social Science Computer Review, vol. 27, no. 4, pp. 524-538.
- Lorenz, J., Rauhut, H., Schweitzer, F. and Helbing, D., 2011.
How social influence can undermine the Wisdom of Crowd effect.
Proceedings of the National Academy of Sciences, vol. 108, no. 22,
pp. 9020-9025.
- Neis, P. and Zielstra, D., 2014. Recent Developments and Future
Trends in Volunteered Geographic Information Research: The Case of
OpenStreetMap. Future Internet, vol. 6, no. 1, pp. 76-106.
- Schenk, E. and Guittard, C., 2011. Towards a characterization of
crowdsourcing practices. Journal of Innovation Economics, vol. 7,
no. 1, pp. 93-107.
- Stranders, R., Ramchurn, S.D., Shi, B. and Jennings, N.R., 2011.
CollabMap: Augmenting Maps Using the Wisdom of Crowds.
- Surowiecki, J., 2004. The Wisdom of Crowds: Why the Many Are
Smarter Than the Few and How Collective Wisdom Shapes Business,
Economies, Societies and Nations. New York: Doubleday books.
- Vukovic, M., 2009. M. Crowdsourcing for Enterprises.
- Wu, G., Harrigan, M. and Cunningham, P., 2011. Characterizing
Wikipedia pages using edit network motif profiles.
- Zhao, Y. and Zhu, Q., 2014. Evaluation on crowdsourcing
research: Current status and future direction. Information Systems
Frontiers, vol. 16, no. 3, pp. 417-434.
BIOGRAPHICAL NOTES
Talia Dror is a PhD student in Mapping and Geo-Information
Engineering at the Technion – Israel Institute of Technology.
Dr. Sagi Dalyot is a faculty member at the Mapping and
Geo-Information Engineering at the Technion – Israel Institute of
Technology. Since 2011, Dr. Dalyot acts as Vice Chair of Administration,
FIG Commission 3 on Spatial Information Management. His main research
interests are geospatial data interpretation and integration,
participatory mapping, LBS, and citizen science.
Prof. Yerach Doytsher graduated from the Technion – Israel
Institute of Technology in Civil Engineering. He received a M.Sc. and
D.Sc. in Geodetic Engineering also from Technion. Until 1995 he was
involved in geodetic and mapping projects and consultations within the
private and public sectors in Israel and abroad. Since 1996 he is a
faculty staff member in Civil Engineering and Environmental at the
Technion. He is the Chair of FIG Commission 3 on Spatial Information
Management for the term 2011-2014, and is the President of the
Association of Licensed Surveyors in Israel.
|